LLMs as physician assistants: Hugging Face benchmark testifies for GPT and Co.
The operators of a hosting platform for AI models offer a benchmark to assess the use of LLMs in the healthcare sector.
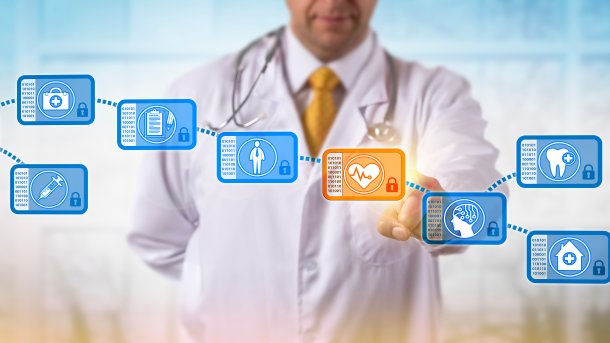
(Bild: Superstar/Shutterstock.com)
The operators of the AI platform Hugging Face have presented the "Open Medical-LLM Leaderboard". The benchmark evaluates large language models (LLMs) according to how well they perform on questions in the healthcare sector. Hugging Face's motivation is that mistakes – LLMs tend to hallucinate – in small talk are of little consequence, but in healthcare a wrong explanation or answer can have serious consequences for patient care or treatment outcomes.
Diagnosis correct, contraindication ignored
As an example, the blog post publishing the benchmark cites a medical question about the care of a pregnant patient complaining of fever, headache and joint pain after being bitten while gardening. A test for Lyme disease is performed, and the question is what medication is best to help the patient. The options are ibuprofen, tetracycline, amoxicillin and gentamicin.
Although the LLM GPT-3.5 reacts correctly to suspected Lyme disease, it selects the active ingredient tetracycline, for which there is a clear contraindication for use during pregnancy. GPT-3.5, on the other hand, claims that it is safe to take after the first trimester of pregnancy.
(Bild: Hugging Face)
According to Hugging Face, a benchmark is therefore essential to be able to assess the extent to which LLMs can be used in the healthcare sector.
Medical data sets as a basis
The benchmark uses numerous medical datasets, including MedQA (USMLE) (Medical Domain Question Answering), PubMedQA, MedMCQA (Medical Domain Multiple-Choice Question Answering) and parts of MMLU (Measuring Massive Multitask Language Understanding) for questions relating to medicine and biology. The leaderboard evaluates the medical knowledge and the ability of the individual models to answer specific questions.
(Bild: Hugging Face)
The accuracy of the answers (Metric Accuracy, ACC) is the main factor for evaluating the models. The leaderboard uses the open-source framework Eleuther AI Language Model Evaluation Harness to evaluate the large language models.
Further details, including the individual data sets, can be found on the Hugging Face blog. The article contains an interactive table with the results of some language models.
(rme)